Healthcare leaders worldwide are making big moves in AI. 85% of them plan to invest in generative AI over the next three years. This shift shows how AI trends are changing healthcare. The telehealth sector proves this momentum—its market value will jump from $79.93 billion in 2023 to $290.90 billion by 2032.
Bask Health sees how AI is changing healthcare through better diagnoses, customized treatment plans, and improved patient tracking. Patient monitoring from afar has become the main goal, and 41% of healthcare leaders will implement AI solutions in this area soon. AI algorithms now analyze patient data to spot health risks early, which makes them vital for managing chronic conditions.
This piece gets into AI's current role in healthcare, from implementation blueprints to diagnostic systems and monitoring solutions. You'll learn about tech advances that are changing patient care and healthcare delivery.
Key Takeaways
- AI is a Game-Changer: 85% of healthcare leaders are investing in AI, with telehealth set to hit $290.9 billion by 2032.
- Smarter Diagnostics: AI-powered imaging can detect diseases with 90%+ accuracy, improving early detection and treatment.
- Predictive Healthcare: AI-driven analytics help forecast patient risks and optimize care, reducing hospitalizations and costs.
- Remote Monitoring Revolution: Wearable AI-powered devices track real-time health data, enabling proactive patient care.
- Automation Boosts Efficiency: AI reduces admin workload by 45%, saving time and cutting hospital costs by millions.
- Integration Challenges Exist: Legacy systems slow AI adoption, but smart data migration and cloud-based solutions are bridging the gap.
- AI is the Future of Healthcare: With rising adoption and proven ROI, AI isn’t just an option—it’s the new standard in healthcare.
Current State of AI in Healthcare
Machine learning technology leads the AI healthcare market share in 2023. At Bask Health, we see AI becoming part of healthcare systems to support clinical decisions and streamline processes.
Key AI Technologies Driving Change
Healthcare relies on three basic AI technologies:
- Machine Learning: This helps analyze big healthcare datasets to diagnose and predict diseases
- Deep Learning: We use this to process medical images and complex patient data
- Natural Language Processing: This helps manage clinical documents and patient communication
AI algorithms now match or outperform humans in certain diagnostic tasks. Recent research shows how AI can spot melanoma through deep convolutional neural networks. These networks analyze images by looking at pixels and disease labels directly.
Market Size and Growth Projections 2024
The global AI healthcare market reached $19.27 billion in 2023. Experts predict it will grow at a CAGR of 38.5% through 2030. Healthcare organizations see great returns on their AI investments. They earn $3.20 for every dollar spent and see returns within 14 months.
Microsoft-IDC's newer study, published in March 2024, shows that 79% of healthcare organizations now use AI technology. The market should hit $187.7 billion by 2030. This growth comes from the need to boost efficiency, accuracy, and patient outcomes.
Software solutions dominate the market with 46.3% of the global share. Care providers, payers, and patients widely adopt AI-based software. The FDA has approved almost 1,000 AI/machine learning-enabled medical devices, and submissions keep growing exponentially.
AI Implementation Framework for Hospitals
Bask Health knows that hospitals need a well-laid-out framework to implement AI. This framework must cover infrastructure, security, training, and finances. Our experience proves that AI adoption succeeds with proper planning and execution.
Technical Infrastructure Requirements
Hospitals need strong technical foundations before implementing AI. The basic components should include:
- High-performance computing systems for data processing
- Flexible storage solutions for large datasets
- Strong network infrastructure with sufficient bandwidth
- Edge computing capabilities for up-to-the-minute processing
- Integration platforms for existing hospital systems
Data Security and HIPAA Compliance
Data privacy is a crucial component of healthcare AI implementation. Organizations must set up strict security measures to protect Protected Health Information (PHI) with AI technology. Healthcare providers should ensure their AI systems follow HIPAA regulations through:
- Data encryption for both stored and transferred information
- De-identification of patient data using safe harbor methods
- Regular security audits and risk assessments
- Strict access controls for AI systems
Staff Training and Change Management
Staff education and change management determine the success of AI implementation. Research shows that healthcare staff's varying AI literacy levels create a major barrier to implementation. Organizations also struggle because faculty and curriculum leaders lack basic AI knowledge.
Cost Analysis and ROI Metrics
Our detailed ROI analysis shows that healthcare organizations can expect positive returns yearly from AI investment. Organizations earn $3.20 for every dollar invested in AI technology. They typically see ROI within 14 months. The cost savings are impressive:
- $1,666.66 per day per hospital in diagnosis during the first year
- $17,881 per hospital in diagnosis by the tenth year
- $21,666.67 per day per hospital in treatment during the first year
- $289,634.83 per day per hospital in treatment by the tenth year
These numbers show substantial financial benefits through workflow improvements, increased efficiency, and better diagnostic accuracy. Reduced waiting times, improved triage processes, and faster patient care drive this positive ROI.
AI-Powered Diagnostic Systems
Medical imaging analysis and disease detection are the lifeblood of AI's effect on healthcare diagnostics. Bask Health's data shows that hospitals perform 3.6 billion imaging procedures annually, but about 97% of this data is unused.
Medical Imaging Analysis Tools
AI-powered imaging analysis has made big breakthroughs in many areas. These systems analyze medical scans with amazing precision and boost diagnostic accuracy. Our analysis shows deep learning algorithms have shown remarkable results in:
- Breast cancer detection with 90% sensitivity compared to 78% for radiologists
- Pneumonia identification from chest radiographs with 96% sensitivity
- Melanoma diagnosis matching dermatologist-level accuracy
- Diabetic retinopathy assessment through retinal image analysis
The Food and Drug Administration has approved all but one of 400 AI algorithms for the radiology field. These tools work as powerful assistants that boost radiologists' diagnostic capabilities and workflow efficiency.
Disease Detection Algorithms
Machine Learning (ML) and Deep Learning (DL) algorithms are the foundations of modern disease detection systems. These algorithms excel at pattern recognition and feature extraction, boosting diagnostic accuracy in medical conditions of all types.
Convolutional Neural Networks (CNN) are without doubt the most effective architecture to analyze medical images. CNNs use multiple processing layers to extract both higher and lower-level information from input data that enables precise disease identification.
Our research shows that AI-based diagnostic systems deliver exceptional results in early disease detection. Deep learning algorithms cut false positives by 5.7% and false negatives by 9.4% in breast cancer diagnosis. The technology has also delivered promising results when it spots lung nodules on CT scans and identifies early signs of Alzheimer's disease.
Hospitals save money when they use these diagnostic systems. Those using AI-powered diagnostics save about $1,666.66 per day in their first year. By year ten, these savings jump to $17,881 per hospital in diagnostic costs.
We expect AI diagnostic capabilities to keep advancing. The technology processes so much medical data while staying highly accurate that it's a great way to get better healthcare. Our work at Bask Health shows these systems boost clinical decision-making and improve patient outcomes in medical specialties of all types.
Remote Patient Monitoring Solutions
Remote patient monitoring (RPM) has become crucial to modern healthcare delivery. We drove this change through FDA-cleared devices that enable continuous patient observation beyond hospital walls. Bask Health's RPM solutions work with AI algorithms to create a powerful system that manages healthcare proactively.
Immediate Data Collection Methods
FDA-cleared monitoring devices now capture vital health metrics including:
- Heart rate and heart rate variability
- Blood pressure measurements
- Blood oxygen saturation levels
- Respiratory rate tracking
- Weight fluctuations
- Glucose level monitoring
Healthcare providers receive data from these devices immediately, which allows continuous review of patient's health status. The integration of IoMT (Internet of Medical Things) devices will soon expand these capabilities. This will create thousands of data points compared to single measurements during traditional office visits.
Predictive Analytics for Patient Care
AI algorithms process big amounts of patient data effectively and identify subtle changes that might indicate potential risks. Machine learning models can review hundreds of variables at once, while human clinicians don't deal very well with processing multiple data points.
NYU Langone Health System's Division of Applied AI Technologies (DAAIT) shows the power of predictive analytics. They developed models identifying patients at risk of deterioration and those with potential sepsis. These predictions help healthcare providers modify treatment plans proactively and reduce adverse outcomes.
Alert Systems and Intervention Protocols
Alert systems can overwhelm healthcare providers without proper management. Studies show that 54% of false alerts come from inappropriate measurement techniques, while 37% result from system inefficiencies. Our sophisticated alert protocols address this challenge by:
- Filtering and prioritizing alerts based on severity
- Establishing patient-specific thresholds
- Enabling rapid response protocols
- Aiding immediate communication between care team members
These systems have shown remarkable results. Patients with chronic obstructive pulmonary disease (COPD) using AI-enabled pulse oximeter devices experienced fewer hospitalizations through early detection and intervention. Healthcare facilities that use RPM solutions also report reduced emergency visits and unnecessary healthcare costs.
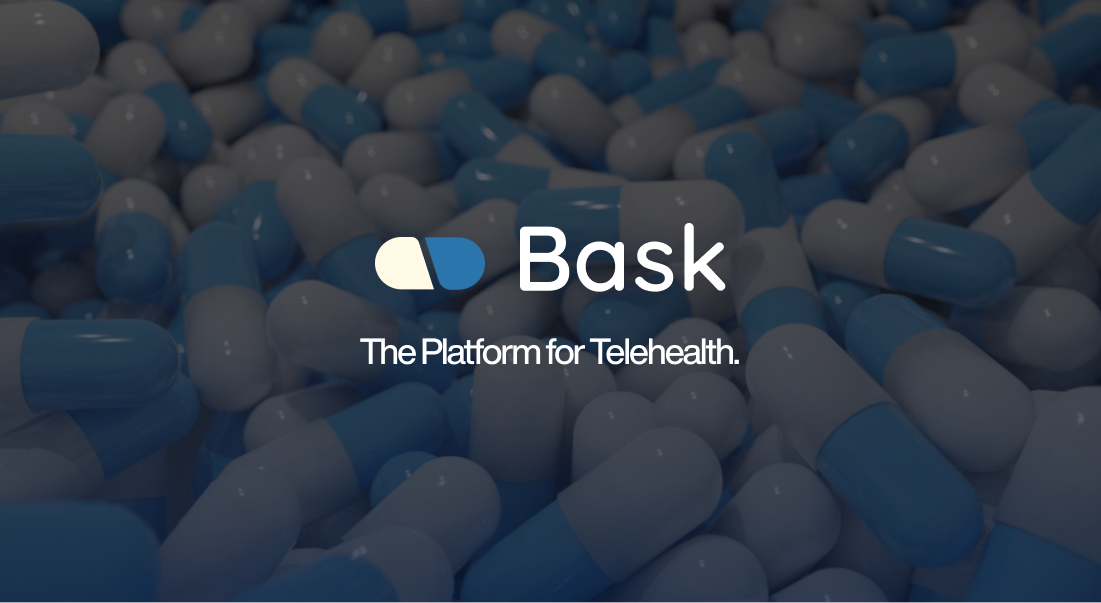
System Integration Challenges
AI implementation in healthcare systems faces its biggest hurdles with integration challenges. Bask Health's data shows that 73% of healthcare provider organizations still use legacy information systems. This creates major obstacles for modern AI integration.
EHR Integration Issues
Electronic Health Record (EHR) systems just need major technical changes to integrate AI. Healthcare organizations struggle with large volumes of integrated data, varying data standards, and interoperability problems.
Research studies and clinical trials face real limitations due to non-standardized terminology and format for Patient-Generated Health Data (PGHD). Healthcare providers must spend extra time reviewing PGHD during patient visits. They don't get paid for this additional work.
Legacy System Compatibility
Legacy systems create operational silos and security weak points that block AI implementation. A HIMSS survey ranks legacy systems as the third biggest security risk for healthcare IT leaders. These outdated systems create several critical problems:
- They don't work well with modern technologies
- They cost too much to maintain and create technical debt
- They slow down operations and disrupt workflows
- They create security risks and compliance issues
- They can't scale or adapt easily
All the same, healthcare organizations can't simply replace legacy systems. The costs run too high and operations could face disruption. Organizations should review their current technology infrastructure and data capabilities before adding AI technologies. This helps them spot gaps they must address.
Data Migration Strategies
Data migration needs careful planning and execution. Traditional data migration has its challenges, but AI adds smarter features and flexibility to the process.
AI helps data migration through smart pattern recognition and automated validation. It spots data inconsistencies by comparing current data with historical patterns. AI also helps after migration by matching migrated data against source data. This finds differences without extensive manual checks.
Organizations should think over various migration approaches based on their needs. Large organizations can benefit from a trickle data migration strategy. It lets both source and target systems run at once, which reduces downtime. Complex systems work best with phased migration. This step-by-step approach moves data by departments or locations and lowers the risk of failure.
Conclusion
Bask Health's work shows AI's powerful impact on healthcare delivery, especially with telehealth services. The market will reach $290.90 billion by 2032, and this is a big deal, as it means that our healthcare organizations see massive returns on their AI investments.
Healthcare providers are seeing amazing results. AI diagnostic systems are matching or outperforming human accuracy in specific tasks. Remote patient monitoring solutions now enable proactive care management. The cost savings are impressive—$289,634.83 per day per hospital in treatment costs after ten years of use.
Legacy systems and EHR platforms pose integration challenges. Yet healthcare organizations keep adopting AI technologies because the benefits are clear. The core team needs proper training, data security protocols, and implementation frameworks to integrate AI successfully.
AI will soon become the backbone of healthcare delivery. We'll see machine learning algorithms tackle more complex datasets. Diagnostic systems will become more precise, and remote monitoring solutions will catch issues earlier. AI-powered healthcare isn't just an option anymore—it's becoming the new standard.
Bask Health helps organizations direct these technological shifts. Our unique experience shows that successful AI implementation needs careful planning, resilient infrastructure, and quick adaptation to new technologies.
References
- American Medical Association (AMA). Health care technology trends 2025: AI benefits, wearable use cases, and innovations. AMA Digital Practice Management. https://www.ama-assn.org/practice-management/digital/health-care-technology-trends-2025-ai-benefits-wearable-use-cases-and. Accessed February 13, 2025.
- Grand View Research. Artificial intelligence in healthcare: Market analysis and forecast. Grand View Research. https://www.grandviewresearch.com/press-release/global-artificial-intelligence-healthcare-market. Accessed February 13, 2025.
- American Hospital Association (AHA). How AI is improving diagnostics, decision-making, and care. AHA Center for Health Innovation. https://www.aha.org/aha-center-health-innovation-market-scan/2023-05-09-how-ai-improving-diagnostics-decision-making-and-care. Accessed February 13, 2025.
- BMC Medical Education. Integrating AI into medical education: Challenges and opportunities. BMC Medical Education. https://bmcmededuc.biomedcentral.com/articles/10.1186/s12909-023-04698-z. Accessed February 13, 2025.
- PubMed Central (PMC). AI-driven analytics in patient care: A systematic review. PMC. https://pmc.ncbi.nlm.nih.gov/articles/PMC3885909/. Accessed February 13, 2025.
- Tenovi. AI in remote patient monitoring: Enhancing healthcare outcomes. Tenovi Blog. https://www.tenovi.com/ai-in-remote-patient-monitoring/. Accessed February 13, 2025.
- Leobit. Legacy system modernization in healthcare and pharma: Challenges and solutions. Leobit Blog. https://leobit.com/blog/legacy-system-modernization-in-healthcare-and-pharma-challenges-and-solutions/. Accessed February 13, 2025.
- American Medical Association (AMA). Health care technology trends 2025: AI benefits, wearable use cases, and innovations. AMA Digital Practice Management. https://www.ama-assn.org/practice-management/digital/health-care-technology-trends-2025-ai-benefits-wearable-use-cases-and. Accessed February 13, 2025.